Number Of Slots In Hash Table
Use multiple hash tables with different hash functions. →On insert, check every table and pick anyone that has a free slot. →If no table has a free slot, evict the element from one of them and then re-hash it find a new location. Look-ups and deletions are always O(1) because only one location per hash table is checked. Say we are given keys in the range 0 to 999, and have a hash table of size 10. In this case, a possible hash function might simply divide the key value by 100. Thus, all keys in the range 0 to 99 would hash to slot 0, keys 100 to 199 would hash to slot 1, and so on. Given a hash table with n keys and m slots, with the simple uniform hashing assumption (each key is equally likely to be hashed into each slot). Collisions are resolved by chaining. (a) What is the probability that the first slot ends up empty? (b) What is the expected number of slots that end up not being empty?
- Number Of Slots In Hash Tablespoon
- Number Of Slots In Hash Table Search
- Number Of Slots In Hash Tableau
- Number Of Slots In Hash Tables
Robin Hood hashing is a technique for implementing hash tables. It is based on open addressing with a simple but clever twist: As new keys are inserted, old keys are shifted around in a way such that all keys stay reasonably close to the slot they originally hash to. In particular, the variance of the keys distances from their 'home' slots is minimized.
Key properties include:
- Lookup is O(1) ammortized and O(ln n) in worst case
- Fast also when looking up keys that does not exist
- Minimal variance on distances to 'home' slots
- Cache friendly and memory efficient (no linked lists or additional pointers)
- Performes well under load factors as high as ~0.9
Make sure you have a basic understanding of hash tables in general (see Hash Tables), and of open addressing in particular (see Open Addressing).
Probe Sequence Lengths
The algorithm is based on the notion of probe sequence lengths (PSL). The PSL of a key is the number of probes required to find the key during lookup.
A key with a low PSL can be thought of as rich, and a key with a high PSL can be thought of as poor. When inserting a new key the algorithm moves the rich in favor of the poor (“takes from the rich and gives to the poor”), hence the name Robin Hood hashing.
Insertion
If the slot that the key hashes to is empty, we insert the key there and return. If not, we start probing for an empty slot. When encountering an occupied slot we compare the PSL of the existing key, with the PSL that the new key would have if inserted in that slot. If the new key has a higher PSL it is 'poorer' and it would be unfair to let go on further, so we swap: The new key is inserted, and the existing key is taken out and is now the key to insert. The probing continues until an empty slot is found.
Example: Insertion of key 76 which hashes to the third slot. The PSL for a key is shown below to the right.
Lookup
The simplest strategy is to look for the key in the slot to which it hashes, and if not found, follow the probing sequence. The probing terminates when an empty slot is found, or a key is encountered which has a PSL higher than the sought key would have in that slot. (If the sought key had been in the table, it would have been located before that key.)
Starting in the middle
The probability of finding a key is the slot it originaly hashed to is low. In fact, the probability of finding the key a certain number of steps into the probe sequence is higher. A simple optimization is therefore to keep track of the overall mean PSL, and probe up and down from that value. The sequence to probe would look as follows:
mean, mean − 1, mean + 1, mean − 2, mean + 2, …
This technique is called smart search.
Optimal probe order

The distribution of keys is not uniform around the mean however, so there's still room for improvement. By keeping track of the distributions of the PSLs, one can probe the slots in order of decreasing probability. The technique is called organ-pipe search.
As an example, the original paper on Robin Hood hashing gives the following distribution for a nearly full table:
While this approach improves slightly on the average number of probes, it requires keeping track of the distribution which incures a constant time overhead and a logarithmic memory overhead.
It should also be noted that both smart search and organ-pipe search hop back and forth in memory, so neither of them utilize the cache very well.
Removal
As with normal open addressing, you can't simply clear out a slot, as that could cause future lookups to fail. You can mark slots as deleted (create so called tombstones, see Hash Tables: Open Addressing) but Robin Hood hashing lends itself to an even better technique, called backward shifting.
Backward shifting works as follows: The slot holding the key to remove is cleared out. The algorithm then starts shifting the keys in the following slots back one step to fill the gap. The backward shifting continues until a key is encountered with PSL 0 (since it would would be shifted before the slot it hashes to), or an empty slot is found.
Example: The key 15 is to be removed from the hash table below.
The reason why this works in Robin Hood hashing is because the keys are always sorted according to the index of the slot they originally hash to. Here's an illustration:
A note on tombstones
The original paper suggests using tombstones. While it does provide better performance for removal, it comes with the same drawbacks as when used in standard open addressing. Lookup and insert algorithm gets slightly more complex, and the tombstones causes slightly longer chains (higher PSLs).
In Robin Hood hashing, there's a clever trick to mitigate the performance impact due to longer chains. By keeping track of the global min and max PSL, one can limit the probing to that range. The probing can be started min steps into the sequence, and stop early at the max value.
Probing techniques
Different probing techniques usually provide a trade-off between memory locality and avoidance of clustering. Since Robin Hood hashing is relatively resilient to clustering (both primary and secondary), linear probing—the most cache-friendly alternative—is typically used.
Complexity
The worst case runtime complexity is O(n) for all operations. As usual however, the more interesting analysis is on the expected runtime.
With smart search, or organ pipe search, the expected lookup time is O(1). The expected length of the longest PSL (and thus the expected runtime complexity of lookup, remove and insert) in a full table is Θ(ln n).
With linear probing the variance of all probe lengths is minimized. In a full table the variance is Θ(n) which is in fact optimal among all open addressing techniques that do not look ahead in the table.
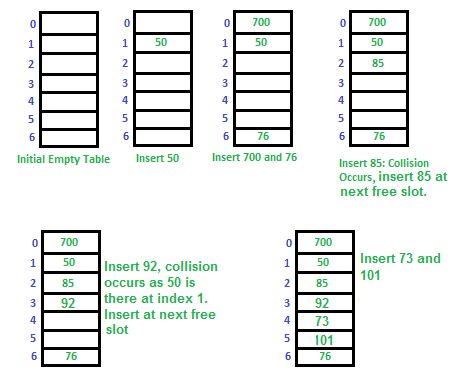
Comments
11.2-1Suppose we use a hash function h to hash n distinct keys into an array T oflength m. Assuming simple uniform hashing, what is the expected number ofcollisions? More precisely, what is the expected cardinality of k and l where k is not equal to l and h(k) = h(l)}
Solution:
α = Expected Number of Collisions = n/m . This is also called the load factor, which means how many keys hashed to 1 slot of the table.
11.2-2Demonstrate what happens when we insert the keys 5; 28; 19; 15; 20; 33; 12; 17; 10 into a hash table with collisions resolved by chaining. Let the table have 9 slots, and let the hash function be h(k) = k mod 9
Solution:
The hash table with collisions resolved by chaining looks like below:
1 ->10->19->28 2 ->20 3 ->12 4 5 -> 5 6 ->33->15 7 8 ->17 9
11.2-3Professor Marley hypothesizes that he can obtain substantial performance gains by modifying the chaining scheme to keep each list in sorted order. How does the professor's modification affect the running time for successful searches, unsuccessful searches, insertions, and deletions?
Solution:
The running time will be affected as follows:Both Successful and Unsuccessful Searches take time O(l) where l = length of the list. As long as load factor is constant, it should not affect the average time complexity of O(1).
Number Of Slots In Hash Tablespoon

Insertions: The insertion operation earlier took O(1) because ecah time a new key was added to the head of the list. But now it would take O(l) where l = length of the list.
Deletions: As long as list is doubly linked list, it should still take O(1) time to delete an element from the table. But if it is singly linked list then it would take O(l) whether or not the list is sorted.
11.2-4Suggest how to allocate and deallocate storage for elements within the hash table itself by linking all unused slots into a free list. Assume that one slot can store a flag and either one element plus a pointer or two pointers. All dictionary and free-list operations should run in O(1) expected time. Does the free list need to be doubly linked, or does a singly linked free list suffice?
11.2-5Suppose that we are storing a set of n keys into a hash table of size m. Show that if the keys are drawn from a universe U with U > nm, then U has a subset of size n consisting of keys that all hash to the same slot, so that the worst-case searching time for hashing with chaining is ѳ(n).
Solution:
Since there are m slots available and the size of Universe(U) > nmThe number of keys in Universe per slot > n
=> U > nm
=> U/m > n
Assume that:
Number Of Slots In Hash Table Search
Number of keys (n) = 100
Number Of Slots In Hash Tableau
Number of slots in hash table (m) = 10
Number Of Slots In Hash Tables
Number of keys in U that hash to 1 slot = U/m = n = 100

From the given information, we know that U/m > n which means that at least n keys hash to the same slot of the table.
Since this implementation is achieved via Chaining, so searching in a list of size n will cost ѳ(n) running time.
11.2-6Suppose we have stored n keys in a hash table of size m, with collisions resolved by chaining, and that we know the length of each chain, including the length L of the longest chain. Describe a procedure that selects a key uniformly at random from among the keys in the hash table and returns it in expected time O(L.(1+1/α)).